Abstract
Photometric stereo leverages variations in illumination conditions to reconstruct surface normals. Display photometric stereo, which employs a conventional monitor as an illumination source, has the potential to overcome limitations often encountered in bulky and difficult-to-use conventional setups. In this paper, we present differentiable display photometric stereo (DDPS), addressing an often overlooked challenge in display photometric stereo: the design of display patterns. Departing from using heuristic display patterns, DDPS learns the display patterns that yield accurate normal reconstruction for a target system in an end-to-end manner. To this end, we propose a differentiable framework that couples basis-illumination image formation with analytic photometric-stereo reconstruction. The differentiable framework facilitates the effective learning of display patterns via auto-differentiation. Also, for training supervision, we propose to use 3D printing for creating a real-world training dataset, enabling accurate reconstruction on the target real-world setup. Finally, we exploit that conventional LCD monitors emit polarized light, which allows for the optical separation of diffuse and specular reflections when combined with a polarization camera, leading to accurate normal reconstruction. Extensive evaluation of DDPS shows improved normal-reconstruction accuracy compared to heuristic patterns and demonstrates compelling properties such as robustness to pattern initialization, calibration errors, and simplifications in image formation and reconstruction.
Learning display patterns
The video shows learning process of display patterns. Every learned patterns excels existing heuristic patterns. DDPS can learn optimal patterns from not only heuristic patterns, but also randomimzed initialization.
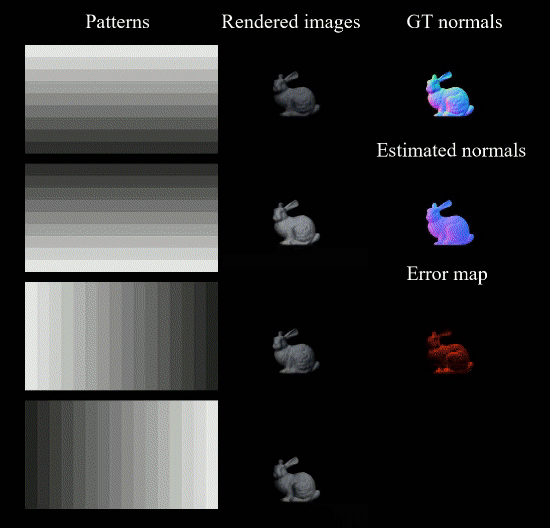
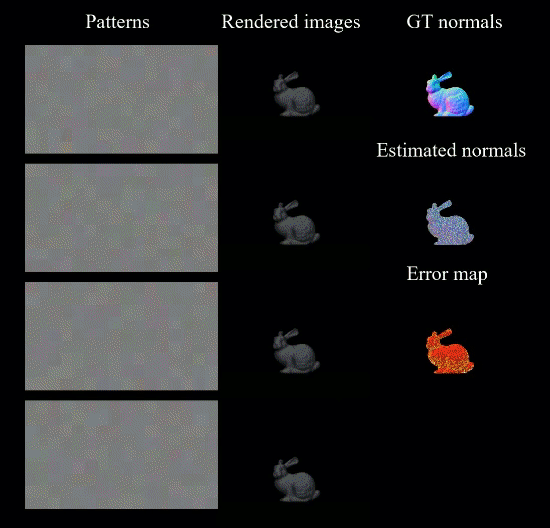
Differentiable framework
Proposed differentiable framework consists of rendering and analytic photometric stereo reconstruction. For differentiable rendering, we adopt Image-based relighting, a weighted sum of basis images. This enables efficient, realistic, and fast rendering.
3D-printed dataset
We creat a real world dataset with known geometry using 3D-printed objects. This dataset leads to not only reduce domain gap between traing and testing stage but also enhance robustness to DDPS’s assumptions.
Results
DDPS shows consistently accurate performance in various real-world objects. We conduct extensive evaluation of DDPS showing its accuracy, generalization and robustness. The extra experiments are enable in main paper and supplemental material.Citation
@article{choi2023differentiable,
title={Differentiable Display Photometric Stereo},
author={Choi, Seokjun and Yoon, Seungwoo and Nam, Giljoo and Lee, Seungyong and Baek, Seung-Hawn},
journal={CVPR},
year={2024}
}